Research
Projects
Publications
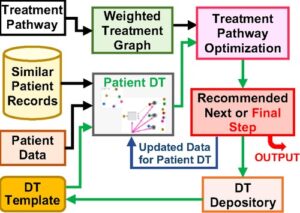
Exploring approaches for predictive cancer patient digital twins: Opportunities for collaboration and innovation
Through the integration of credible multi-source data, we could create connections among the bio-entities, authors, articles, affiliations, and funding.

Building a PubMed knowledge graph
Through the integration of credible multi-source data, we could create connections among the bio-entities, authors, articles, affiliations, and funding.
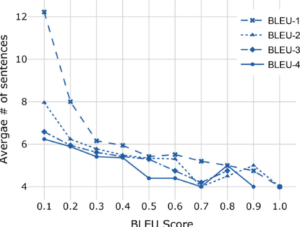
Prior Knowledge Enhances Radiology Report Generation
In this work, we propose to mine and represent the associations among medical findings in an informative knowledge graph and incorporate this prior knowledge with radiology report generation to help improve the quality of generated reports.
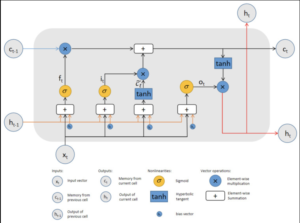
Tracing and forecasting metabolic indices of cancer patients using patient-specific deep learning models
We develop a patient-specific dynamical system model from the time series data of the cancer patient’s metabolic panel taken during the period of cancer treatment and recovery.
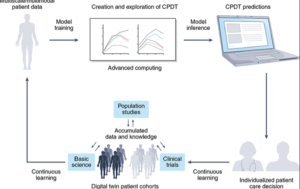
Digital twins for predictive oncology will be a paradigm shift for precision cancer care, Nature Medicine
Cancer patient digital twins (CPDTs) use emerging computing and biotechnologies to build in silico individual representations that dynamically reflect molecular, physiological and lifestyle status across different treatments and time.